AI Machine Learning in IoT Security: Techniques and Tips
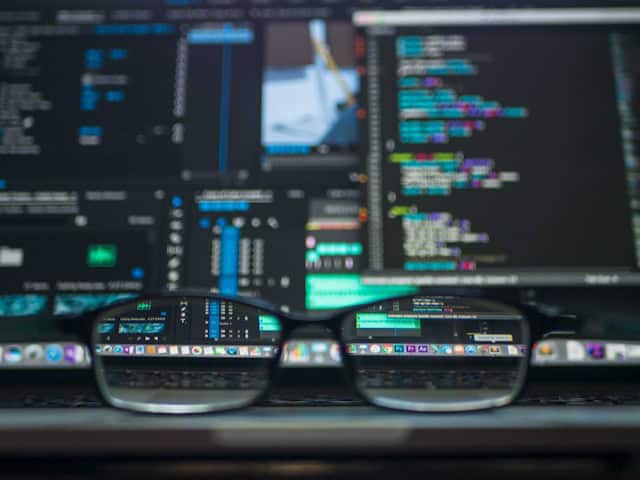
With the steady rise of machine learning and AI in Internet of Things applications has come new security concerns. Machine learning has become a vital player in addressing IoT security challenges, operating to elevate the resilience of IoT ecosystems against threats.
In this blog, we’ll be exploring a selection of ways in which machine learning can be applied in IoT security, including the benefits and drawbacks of using artificial intelligence in this way.
How Is Machine Learning Used to Secure IoT?
Machine learning is used in IoT to increase personalisation, optimise resources, and execute predictive maintenance, but what is the role of AI in IoT security?
- Detecting anomalies
Machine learning algorithms are trained to learn IoT device behaviours and interactions via detecting anomalies. The models have the power to detect abnormal activity by using real-time data. In turn, this assists in uncovering and addressing security breaches. It can do this rapidly, saving your business time.
- Identifying malware
Machine learning algorithms are able to analyse device behaviour and network traffic to uncover any unauthorised access attempts and malware infections, amongst other suspicious activities. This can assist in preventing system disruptions before it’s too late.
- Analysing user behaviours
Machine learning is also leveraged to keep an eye on user interactions with IoT devices, helping to detect attempts of unauthorised access and anomalous activities. This helps to protect sensitive data.
- Encrypting data privacy
Another way machine learning can be used in IoT security is through ensuring data security and privacy in IoT systems are secured. Some machine learning algorithms offer encryption to enable calculations on encrypted information. AI can perform anonymisation and de-identification to protect sensitive data and streamline analysis.
- Identifying emerging threats
The fantastic thing about machine learning is that it can detect unusual patterns in real-time, meaning it can action them with suitable responses before they have fully emerged. We think this is a benefit over traditional security procedures, which sometimes find it difficult to handle novel attacks.
- Analysing software vulnerabilities
By exploring vulnerabilities and security problems in IoT device firmware and software, industry experts can operate to fix them before they are deployed.
Benefits of Machine Learning for IoT Security
So, with such advancements in technology, there have to be some benefits, right? Check out the main benefits of using machine learning in IoT security below.
- Enhanced trust
Because machine learning excels in identifying anomalies and unusual patterns in datasets, it is able to detect possible security breaches early, meaning they can be addressed before it is too late. This can enhance your company’s reputation and trust with customers.
- Adaptive learning
As machine learning models can constantly learn from new data, they are able to adapt to evolving threats and better their detection capabilities as time goes on. This adaptability component is critical as cyber threats continue to grow more and more sophisticated.
- Automation
Automation has assisted in various industries so far, from improving customer experience to making business analytics more sophisticated.
By automatically detecting and actioning threats, machine learning can decrease our reliance on human intervention, thus allowing for swift responses to security threats. This works to minimise the overall impact of a potential breach.
- Scalability
As well as being able to adapt to new threats, machine learning is also able to scale. It can effectively analyse data from various devices at once when monitoring each device becomes impractical. Monitoring devices at the same time makes it easier to maintain security across large-scale deployments, which is fantastic news for larger organisations.
Challenges of Machine Learning in IoT Security
In spite of the above benefits, using machine learning in IoT security is not without its potential drawbacks.
- Complexity
Executing advanced AI in IoT security systems can throw in another layer of complexity to the mix. This factor means that organisations must ensure they have the right expertise and infrastructure to develop and deploy these machine learning models as effectively as possible.
- Privacy
Privacy concerns have continuously been linked to the use of AI over the past few years. The data utilised in training machine learning models may include sensitive information, which means strict regulations must be carefully considered.
- Resources
Training and deploying machine learning models can put a lot of demand on resources, which could be a concern for resource-constrained IoT systems.
- Inaccurate data
Machine learning models require a high-quality input to produce a high-quality output. This means that training models on biased or inconsistent data could result in the production of inaccurate predictions as well as inefficient security measures.
The Future of AI and IoT Security
The relationship between machine learning and IoT is undoubtedly set to evolve significantly in the coming years, mostly driven by technological advancements and growing connectivity, as well as the ever-increasing sophistication of cybersecurity threats.
With higher-powered security measures, organisations can start to enjoy more robust security systems. Here are some specific predictions our experts believe we may encounter in the future:
- Better threat detection
We can only expect machine learning algorithms to become more and more advanced, meaning they’ll be able to improve their ability to detect threats in real-time. Get ready for more sophisticated anomaly detection systems that can spot malicious behaviours with greater accuracy.
- Enhanced predictive analytics
The IoT security systems of the future will likely leverage machine learning for predictive analytics, allowing organisations to foresee possible threats based on historical data. A proactive approach will grow more and more advanced to help companies stop incidents before they even occur.
- Edge computing
As edge computing becomes more and more popular, we may expect it to complement machine learning in IoT security. For example, by processing data closer to the source, a device will be able to make quicker security decisions to reduce a data breach risk during transmission.
- More robust security
Federated learning approaches will allow machine learning models to be trained across various devices without sharing sensitive data. This means that federated learning can provide more robust security measures whilst preserving privacy, making it a very appealing option for IoT environments.
- Stronger ethical considerations
As awareness of AI ethical concerns and data privacy grows, businesses will be forced to focus on strict compliance with regulations. For example, future machine learning models will likely incorporate components to ensure adherence to privacy laws as well as ethical standards.
- A call for education
Education and training will become key as machine learning and IoT security become more prominent across sectors. With this in mind, organisations may need to invest in upskilling their workforce in order for these technologies to be effectively leveraged. At the same time, workers will need to understand how to mitigate the dangers associated with AI and how they can fully collaborate with new technologies.
So, that’s a wrap on machine learning in IoT security! Our team has years of experience navigating machine learning and IoT and can support your in-house team with more complex integrations. We’re experts in all things to do with computer programming, from creating AI assistants to explaining how IoT, big data, and the cloud work.
If you think the expert implementation of AI development services would benefit your business, don’t hesitate to get in touch with us today.